Artificial intelligence-assisted medical imaging and the GDPR
26.03.2021
In November we will be hosting a debate on AI use in medicine at our upcoming Life Sciences Summit – AI in the medical sphere.
Register your interest here. |
In December 2020, the European Parliamentary Research Service (EPRS) published a short At A Glance article on the subject of artificial intelligence (AI)-supported medical imaging and its potential application to the triage of COVID-19 patients[1]. The article provided an interesting overview of the potential impact of AI-supported medical imaging, referring to current examples of the technology in use or in development around the world.
As presented in the article, the potential benefits of AI-supported medical imaging technology are hard to argue with. In short, AI-assisted medical imaging could dramatically reduce the time and manpower required to diagnose and predict the likely progression of disease in COVID-19 patients, analysing chest CT scans in 10 seconds where the same task would take a trained radiologist 15 minutes. Similar benefits could be delivered in relation to other medical conditions which can be analysed using medical imaging techniques such as CT scans and X-ray photography.
The EPRS article also provides a brief overview of the policy and legal challenges that the development and use of machine learning (ML) algorithms for AI-assisted medical imaging may pose in the European Union. Those same challenges are highly likely to apply equally in other jurisdictions such as the UK and the US which have mature data protection and product liability regimes.
Policy and legal challenges for AI-assisted medical imaging
ML algorithms need to be trained using large, high-quality patient data sets in order to ensure accuracy and reliability. The EPRS article discusses the difficulty of obtaining and curating such data sets in the first place. These difficulties are said to be twofold: first, there is a real lack of historic data available on the long-term impacts of new and emergent diseases such as COVID-19, which hinders the development of algorithms capable of identifying early markers of a long term prognosis; second, there is a lack of consistent and representative data available on which to train ML algorithms, due to a variety of factors including regional differences in scanning techniques and the absence of standardised protocols for the training and validation of machine learning algorithms.
This is the policy challenge – how should policymakers ensure that large, high-quality patient datasets are made available to ML algorithm developers in order to take advantage of AI-assisted medical imaging technology as quickly as possible? We don’t claim to have an answer, but this question does set the scene for the legal challenges posed by the development of ML algorithms for use in the medical sector.
The author of the EPRS article expresses the view that “under the General Data Protection Regulation, patients must give prior informed explicit consent for the use of their medical scans and imaging data in developing an AI algorithm, and this must be renewed before the design and training of each new version”. The author goes on to question whether obtaining such consent is really plausible given the time pressure under which these ML algorithms are developed. This is one of the legal challenges (our views on the other legal challenges raised by the EPRS article can be found here).
Particularly where consent is sought to process data about a person’s health, which is special category personal data, the circumstances in which consent is deemed to have been validly given under the GDPR are highly circumscribed. As a result, it certainly does not seem plausible to obtain the consent of every patient each time a new ML algorithm is developed using a data set containing their data, especially as such data sets might contain information from hundreds or even thousands of individual patients. However, the GDPR provides some potential alternative legal routes for ML algorithm developers to use training data sets containing patient health data without having to rely on their consent.
Getting around the consent challenge
Under the GDPR, a lawful basis is required for the processing of personal data, of which consent is one. If the personal data being processed is so-called special category personal data (which includes information about a person’s health), then the processor must rely on an additional lawful basis. Explicit consent is one such additional lawful basis. The additional lawful bases for processing special category data are much more specific and restrictive, but as a result it is generally true that if an additional lawful basis is satisfied, then one of the less exacting lawful bases for processing ordinary personal data will also be satisfied.
Consent is only one of the lawful bases under the GDPR for the processing of personal data, including special category personal data. Another additional lawful basis for processing special category personal data exists where such processing is “necessary for […] scientific or historical research purposes”, subject to that processing being based on European Union or national law and there being suitable safeguards in place to protect the individual rights and freedoms of data subjects.[2]
“Scientific research purposes” could easily encompass research and development in the field of machine learning for medical diagnostic and analysis purposes, even where that research and development is conducted with commercial gain in mind. Meanwhile, appropriate (though not necessarily sufficient) safeguards would include pseudonymisation of the personal data being processed and the use of encryption to protect it from unauthorised access.
The circumstances in which data processing for scientific research “is based on” national law obviously varies from country to country. Under UK law, which continues to implement a version of the GDPR which is materially identical to that under EU law despite Brexit, scientific research data processing is based on UK law where it meets the requirements of the GDPR and is also “in the public interest”. The UK data authority considers that research is in the public interest where there is “a benefit to the wider public or society as a whole, rather than to [the researcher’s] own interests or the interests of [a] particular individual.”[3] Research into ML algorithms which deliver a public good, namely faster and more effective diagnosis and prognosis of patients suffering from disease, is highly arguably in the public interest.
Therefore it is entirely plausible that ML algorithms could be trained on personal data without patient consent in the United Kingdom, using the scientific research lawful basis found in the GDPR instead. To the extent that they take largely the same approach to the scientific research lawful basis as the United Kingdom, the same can also be said of the Member States of the European Union.
Of course, data protection and the GDPR are separate from the patient confidentiality obligations which exist in the United Kingdom. In the United Kingdom and other jurisdictions which have a similar concept of patient confidentiality, patient consent may still be required before their confidential information can be used in research, even if the researcher can rely on a lawful basis other than consent for processing their personal data.
Postscript: could the GDPR lawful bases be irrelevant in certain circumstances?
A data processor such as an ML algorithm developer only needs to demonstrate a lawful basis for their processing if the data they are processing is personal data. “Personal data” is defined by the GDPR as “any information relating to an identified or identifiable natural person”.[4] Therefore, if the patient to whom the information in a training dataset relates is not identifiable because the training dataset has been anonymised, the ML algorithm developer is free to process the information without consent or any other lawful basis. This would completely avoid the GDPR compliance difficulties raised by the author of the EPRS article.
It should be borne in mind that true anonymisation which is sufficient to take personal data out of the scope of the GDPR is exceptionally difficult to achieve. To be anonymised, personal data must be stripped of all the elements that identify the data subject, and there must be no means reasonably available that would allow the data subject to be re-identified.[5] That is easier said than done. However, if an effective ML algorithm training dataset can be built using anonymised data and therefore avoid the need for a lawful basis under the GDPR whenever it is used, that dataset will be significantly easier from a legal perspective for ML algorithm developers to use.
———————
[1] M. Kritikos, ‘What if artificial intelligence in medical imaging could accelerate COVID-19 treatment?’ PE656.333, EPRS Scientific Foresight Unit, December 2020.
[2] Art. 9(2)(j) Regulation (EU) 2016/679 (GDPR)
[3] https://ico.org.uk/for-organisations/guide-to-data-protection/guide-to-the-general-data-protection-regulation-gdpr/special-category-data/what-are-the-conditions-for-processing/#conditions10
[4] Art. 4(1) Regulation (EU) 2016/679 (GDPR)
[5] Information Commissioner’s Office, ‘What is personal data? https://ico.org.uk/for-organisations/guide-to-data-protection/guide-to-the-general-data-protection-regulation-gdpr/what-is-personal-data/what-is-personal-data accessed 20 January 2021.
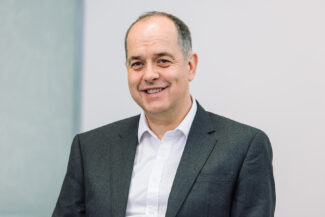
Mark Watts
Author
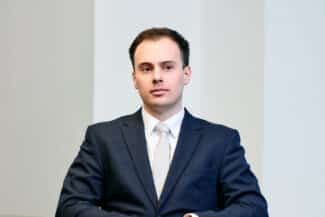
Jamie Hatzel
Author