Artificial Intelligence: some practical risks, challenges and limitations in applying AI
24.10.2017
In this second article of our series on AI and the law, we set out some of the key risks, challenges and limitations to consider in the practical application of AI.
Most of the commentary on AI inevitably focuses on the potential transformative effects the technology will have on business and society. That is to be expected, since it appears that AI remains on or around the ‘peak of inflated expectations’ on the AI hype cycle1 (we look forward to the ‘slope of enlightenment’ phase at which most new technologies find their broadest and most productive use). As we mentioned in our first article in this series, we at Bristows are AI advocates and we believe the technology has the potential to improve business and society. At the same time, we are clear that the only way that AI will fully realise that potential is if the risks inherent in AI systems are properly understood and managed. In this article, we try to set out the key practical risks in applying AI in business and society – in particular, what can go wrong in deploying AI for a particular purpose and what might stop that purpose being fully realised. As such these can be classed as ‘application risks’ as distinct from legal risks, which will be the subject of future posts in this series.
Bad Data: A key feature of many AI systems is how they process and apply large data sets in order to solve problems or execute tasks. Accordingly, the outcome achieved by an AI system will only ever be as good as the quality of data fed into it on which it bases that outcome. How can we be sure that the quality of data inputted into the AI system is ‘good’ enough to deliver the expected outcome? There are many variables here: are the data sets ‘big’ enough, is ‘real-world’ data being used, is the data corrupt, biased or discriminatory? The risk is especially acute for ‘machine learning’ AI which are trained on data inputs and improve outcomes over time based on more and more data being fed to them. Consider the prospect of AI algorithms used to assess likelihood of recidivism amplifying racial biases as has been alleged against an AI tool being used by Durham police force2, or social media AI bots morphing into “Hitler-loving, feminist-bashing troll[s]” having ‘machine learned’ such themes from user engagement.3 (Clearly there are other data-related issues and risks; we will be focusing on data privacy and AI in a future dedicated post in this series.)
Transparency: One of the features of some types of AI is a difficulty in scrutinising how they work, how they decide on a course of action or how they have reached a decision. Sometimes this is because the AI system is opaque by nature; other times the AI system is proprietary and the owner is not willing to open it up to such scrutiny.4 This lack of transparency is commonly referred to as the ‘black box’ problem. How can users trust in AI-delivered outcomes delivered if the inner workings of the system are not easily interpretable by a human? From our own perspective, how can we as lawyers “own” an “answer” to a legal question if we cannot work out how that answer has been derived? Consider that the developers of ‘AlphaGo’, Google DeepMind’s system that defeated the human world champion of the board game ‘Go’, could not explain why their system made some of the complicated moves it did. Organisations need to be accountable for decisions that affect us, especially negative affects where those who have suffered loss should have a route of recourse readily available, and if using ‘black box’ AI does not reinforce accountability then it is difficult to see how such organisations can be confident in using AI or, if they do use it, that the general public will be comfortable in permitting such invisible decision-making. This may impact upon the speed of classification and standardisation of certain applications of AI. If the autonomous vehicle industry is going to be reliant on AI for its driverless cars and requires safety certification from regulators, if the regulators do not see what is going on and the manufacturers are not willing to divulge workings, then how can any classification/standardisation take place?
Misuse: The ‘black box’ problem described above means it may be difficult to determine when it is appropriate to use AI and when it is not. If an AI system is not transparent, it is probably unpredictable to some degree, making it hard to assess what might go wrong, when it might go wrong and the (potentially scaled-up) adverse effects failure might have. It is key for organisations to understand the environment in which AI deployment will take place, to try to conduct a risk assessment, and then get comfortable with those risks. Testing in a non-live environment is to be encouraged. Even then, it may not be possible to test for every potential failure scenario.
Misappropriation: A problem linked to bad data causing bad outcomes is that malicious data could cause malicious outcomes. An AI system designed to have ostensibly positive or, at worst, benign effects could be used for opposite or conflicting purposes – an AI system analysing trends in the energy market could be used to disturb or even disable that market. The risk is amplified when it is considered that AI systems will typically be hosted in the ‘cloud’ or otherwise internet-enabled, opening them up to cybersecurity issues and hacking – if this occurs would the owner or user even know such misuse was happening (let alone individuals affected by the AI’s decisions)? Several recent high-profile cybersecurity attacks only became known by the affected party months after the hacking took place. It is noteworthy that one of the benefits of using AI involves scale. The benefits of applying decentralised, disaggregated AI systems processing more data than could be analysed by humans or conventional IT systems could be offset by the malevolent effects that such a system falling into the wrong hands could wreak.
These are just some of the practical risks we think are involved in applying AI. The size and importance to be given to each risk will differ depending on the type of AI system and the scale and scope of the use case for it. Nevertheless, a sensible consideration of applying AI will take these into account and seek to insert controls to obviate or mitigate the risk in question.
So far in this series, we have set the scene for the AI debate and, in this post, examined some practical risks and issues in deploying AI. In our next post, we will try to set out how society and business might seek to manage some of these risks.
________________________________________
[1] https://www.gartner.com/technology/research/methodologies/hype-cycle.jsp
[2] http://www.bbc.co.uk/news/technology-39857645
[3] http://www.techrepublic.com/article/why-microsofts-tay-ai-bot-went-wrong/
[4] Admittedly this issue is not limited to AI and is equally applicable to algorithms more generally.
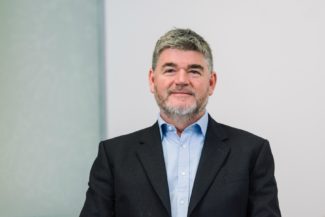
Chris Holder
Author