Artificial Intelligence: how to manage the risks in applying AI
27.10.2017
In this third article of our series on AI and the law, we explore how industry and society typically seeks to manage risks posed by new technologies, how these approaches might apply to AI, and some of the risk management steps industry can take today.
Almost any time a new technology arises, questions are asked about how it will be regulated. Occasionally, the nature of that new technology leads to broader questions of ethics and morality, with regulators encouraged to place those ethical and moral issues ahead of technical progress. An example of this can be found in the field of genomics which saw its early development checked by ethical concerns. AI follows in a long line of new technologies that raise such questions and, as we mentioned in our first article in this series, is attracting close scrutiny from regulators, ethicists and policymakers the world over. In our previous post in this series, we examined some of the key AI risks and issues that will need to be properly understood, managed and mitigated if AI is to realise its potential to the fullest extent; in this post, we will suggest how we, as a society experienced in the adoption of new technologies, might manage those risks as the adoption of AI progresses.
Societies have available to them a set of tools that can be deployed to manage risks in new technologies like AI. Some of these tools are internal to a particular product/service, business or industry; some are external controls imposed by regulators, politicians and courts. When the internal tools do not adequately manage the risks in the new technology, it is more likely that regulators will step in and impose external controls and, in some cases (such as in the early days of genomics), prohibitions. This correlation means it is important for the AI industry – comprising a broad spectrum of developers, service providers and users – to lead the way in developing best practice to address inherent risks without stifling innovation.
One way the AI industry can lead the way is in establishing and implementing industry standards and codes of practice that seek to manage AI risks, for example those that arise in respect of health and safety, data privacy and cyber security. This approach could work on a product-by-product basis, where a producer can apply for a form of certification or accreditation if its product meets the relevant product standard or code. Or it could work on a wider level, where an organisation seeking to operate in the AI space must meet certain minimum entry criteria to join an industry association which helps to foster trust in its members’ AI products and services.
It is possible, indeed likely, that this form of self-regulation will be sufficient for some applications of AI. However, for those applications that involve greater risk, it may be that lawmakers will feel the need to deploy a range of regulations, restrictions and even prohibitions on their use. As we discussed in our previous post, a feature of many AI systems is a lack of transparency (so-called ‘black boxing’), making it difficult to assess the risks posed by the AI system because it is hard to see how risk can be adequately assessed if the inner workings or inner logic of a computer system are not visible.
When such AI systems are applied to, for example, safety-critical infrastructure, or outcomes that could impact on human rights or wellbeing, it is plausible that lawmakers will consider self-regulation to be insufficient and feel obliged to intervene. Such intervention could amount to onerous top-down regulation that seeks to limit such risks but has the unintended but foreseeable effect of stifling development or take-up of AI technology. In more extreme cases, it could amount to blanket bans on certain AI technologies, or possibly just their application in certain realms (see aforementioned examples of safety-critical infrastructure and human rights).
This is not to say that AI is free from legal or regulatory oversight today. We will in future posts in this series touch on how existing legal regimes apply to AI, for example in the fields of liability and data protection, and how they may need to develop to take account of the specific issues raised by some of the unique features of AI. The main point we seek to make in this post is that there is action AI operators can undertake now to take account of the risks, and in so doing get out ahead of the lawmakers, thereby pre-empting the need for top-down regulation and the potential ‘chilling’ effect on innovation that this may bring.
The industry – and by that we mean both developers and users – can develop and adopt key principles to regulate AI in a way that aligns with good governance, best practice and existing legal regimes, in order to ensure it is in the best possible position to meet the concerns of regulators and policymakers going forward. In relation to governance, it is important to have decision-making processes that guide an organisation through the AI deployment and is auditable and understandable at every level. In designing and deploying AI, it is important to meet high performance and quality standards to help ensure that the AI system delivers the intended outcomes and is less likely to mis-align with best practice or breach existing laws.
In considering legal risk, it is important to ensure the design and use of the AI system meets high levels of legal compliance (for example, that the way the AI uses personal data is in compliance with data protection legislation) and that risk management assessments are conducted to understand and mitigate any potentially adverse impacts of the system going wrong. These are just some of the building blocks that can be used to inform a set of core principles that can underpin an organisation’s deployment of AI.
So far in this series, we have set the scene for the AI debate, examined some of the practical risks and issues involved in AI, and, in this post, set out how society and business might seek to manage those risks as the market develops. In our next few posts, we will turn to exploring how our existing legal regimes and insurance models apply to AI today and how they might need to adapt to regulate the market over time.
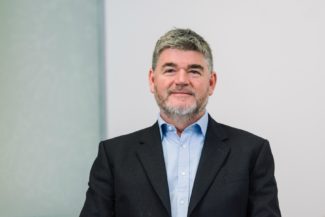
Chris Holder
Author